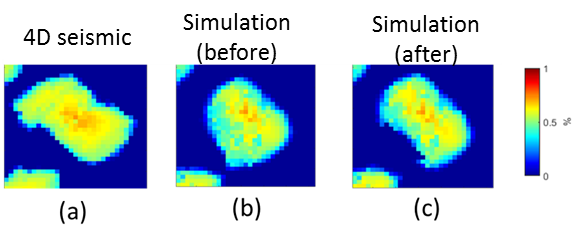
4D seismic is a tool for reservoir monitoring. Through the repetition of seismic surveys during the production of the field it is possible to identify changes that occurred due to the production, as pressurized regions, areas flooded by water injection, etc.
The spatial information (maps of changes) provided by 4D seismic data are extremely important for updating simulation models as they are complementary to well data (fluid rates and pressure) traditionally used in the history matching process. While well data are rich temporarily (usually monthly measurements) and poor spatially (location of wells), 4D seismic data are usually poor temporally (some surveys over the life of the field) but rich spatially (maps in the extension of the fields). Thus, the great value of 4D seismic is related to the ability of this tool to provide information between the wells. For instance, this information may help locate bypassed hydrocarbons, optimize infill drilling and manage injection, impacting considerably on the field management.
4D seismic data can be integrated with simulation models qualitatively and quantitatively. In both cases the aim is to adjust the simulation model so that it generates responses with similar behaviors to the ones observed in 4D seismic (pressure and saturation changes). In the qualitative approach the simulation models updating is manual and 4D seismic data are used as a guide for adjusting the model attributes such as fault transmissibility and permeability. In the quantitative approach, the seismic data are included in the objective function of an assisted history matching (or automatic) and the matching procedure is performed by optimization algorithms.
Although the capacity of 4D seismic to improve reservoir models is well known, there are still many challenges especially concerning the quantitative data integration. In this sense, the research developed in UNISIM is focused on the quantitative use of 4D seismic to update the simulation models aiming the reduction of reservoir uncertainties. We also propose different forms of integration between these two types of data to get the most of them, including unconventional approaches such as the use of engineering constraints to assist the interpretation of geophysical data.